THE Deep Learning is a specialized form of Machine learning which uses neural networks with many layers. Machine learning, on the other hand, often works with simpler algorithms such as decision -making trees or linear models. The deeper structure of the network allows Deep Learning to recognize more complex models in large amounts of data.
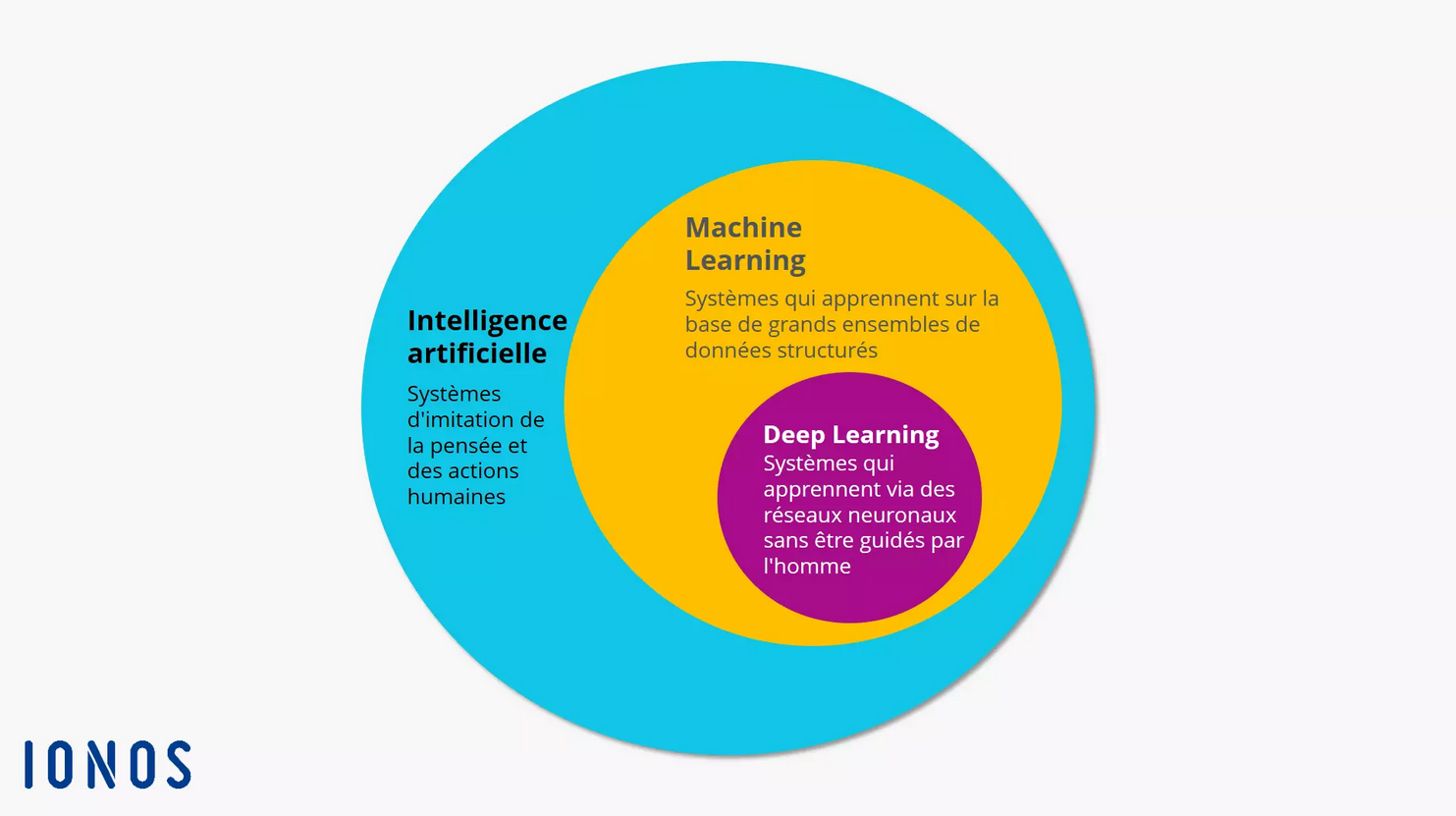
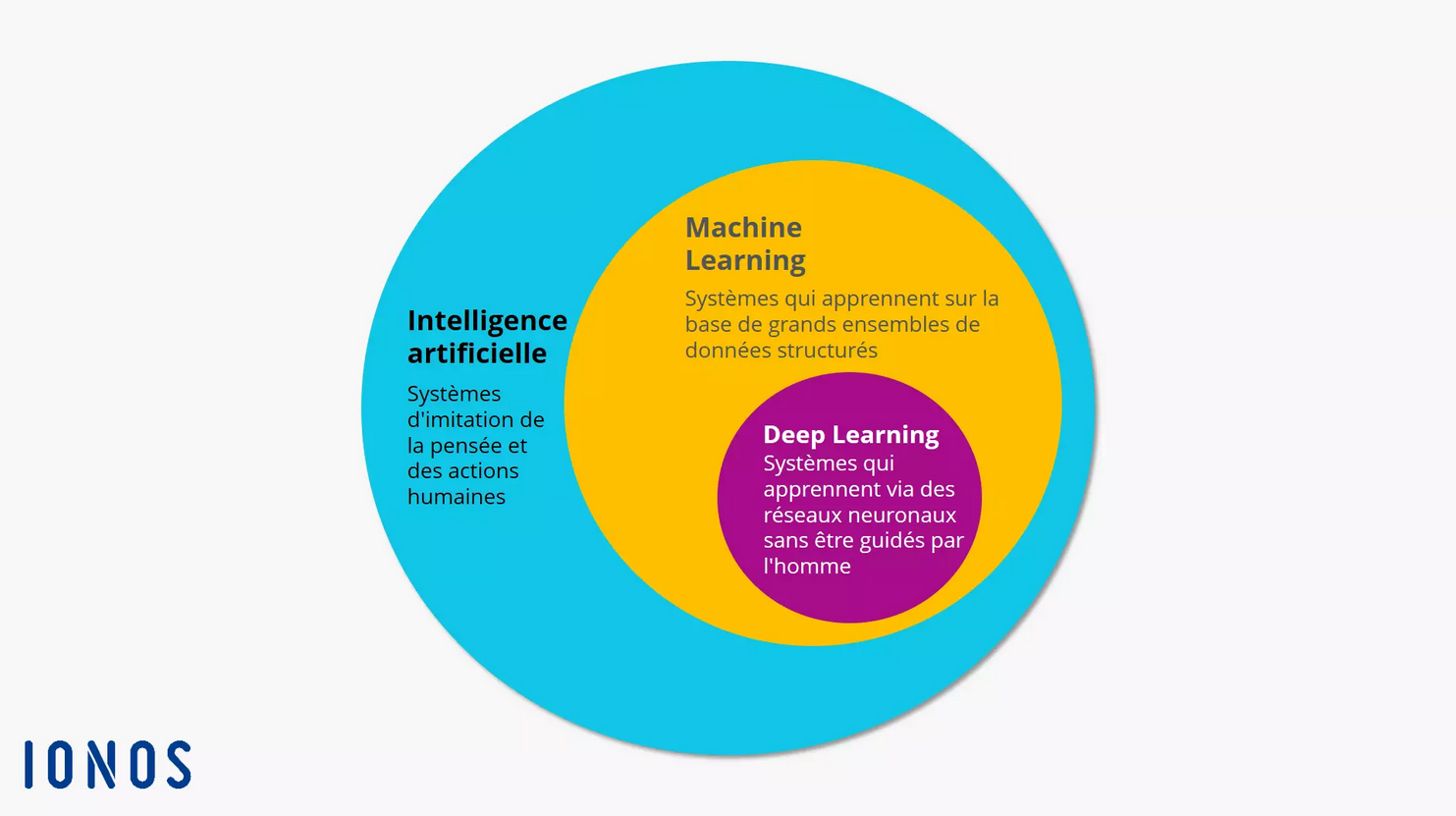
Machine Learning and Deep Learning are part of artificial intelligence. These approaches both have the result of giving computers the ability to make intelligent decisions. However, Deep Learning is a subcategory of machine learningbecause it is based on unattended learning.
In both cases, intelligence is limited to specific uses. We are talking about weak artificial intelligence, as opposed to a strong artificial intelligence which would be able, in many areas and circumstances, to make intelligent decisions similar to those of a human.
Deep Learning vs Machine Learning: What are their differences?
THE Machine learning is an older and simpler technology. It relies on an algorithm that itself adapts the system From the feedback made by humans. The implementation of this technology implies the existence of organized data. The system is then powered by structured and categorized data, which allows it to classify new similar data. Depending on this ranking, the system then performs the programmed actions. He knows how to identify if a photo shows a dog or a cat and classify the document in the corresponding folder.
After a first phase of use, the algorithm is optimized from the developer feedback, which informs the erroneous classifications system and indicate the good categories.
THE Deep Learning does not need structured data. It works from several layers of neural networks, which combine different algorithms Inspired by the human brain. Thus, the system is capable of working from unstructured data.
This approach is particularly suitable for complex tasks, when all aspects of the objects to be treated cannot be categorized upstream. The Deep Learning system itself identifies the discriminating characteristics. In each layer, he is looking for a new specific criterion of the object, which serves as Base for deciding the classification retained for the object at the end of the process.
Important: with Deep Learning, the system itself identifies the discriminating characteristics of the datawithout the need for prior categorization. The system does not need to be driven by a developer. He himself evaluates the need to modify the classification or to create unpublished categories according to the new data.
While machine learning works from a controllable database, Deep Learning needs a much larger data volume. The system must have Several million entries To give reliable results, although this number can vary depending on the task.
In addition, the technology necessary for Deep Learning is more sophisticated. It requires more IT resources and is clearly more expensive than machine learning.
AI Model HUB
Your secure multimodal AI platform
- Compliant with the GDPR and hosted safely in Europe
- Most powerful AI models
- Open Source, without Vendor Lock-in
Summary of differences
Machine learning | Deep Learning | |
---|---|---|
Data organization | Structured data | Unstructured data |
Database | Less bulky | > 1 million data |
Training | Requires human intervention for training | Autonomous learning system |
Algorithm | Modifiable algorithm | Algorithm neural network |
Scope | Simple routine applications | Complex tasks |
Deep Learning VS Machine Learning: areas of application
You can consider machine learning as a precursor technology of the Deep Learning. Concretely, all the tasks performed using machine learning can be resolved with the Deep Learning. The two technologies should therefore not necessarily be opposed.
Deep Learning mobilizes many more resources And is therefore not an efficient process. The fields of application of the two technologies are in principle very distinct: any task that can be performed by machine learning can also be by Deep Learning, but the latter requires greater resources.
For companies, using these technologies represents a enormous competitive advantage, because neither the machine learning nor deep learning are yet the standard in professional daily life.
Different application fields
In the field of Online marketingcompanies often use marketing analysis tools based on machine learning. They assess existing data and can make reliable forecasts on the type of content that leads to conversions, on the content that customers want to read and on the marketing channels that lead to a purchase.
Customer assistance chatbots can also be based on machine learning. They then move towards keywords contained in the request of the user and can guide it to the desired information by asking questions or by proposing options for dialogue. Deep Learning chatbots include the natural language of humans and do not depend on the use of specific keywords. The dialogue is much more effective and the solution offered more relevant.
THE Digital vocal assistants such as Siri, Alexa and Google are now almost always based on vocal synthesis and Deep Learning. The first vocal assistants are also entering businesses. Users can ask them in natural language, for example, to place orders, send emails, create reports or carry out research. Previous systems based on machine learning were unable to perceive the shades of the language and are therefore less well adapted.
In the field of Business Intelligencemachine learning can be used to view the company's key data and make forecasts understandable for human decision -makers. Deep Learning systems in the field of generative AI even allow you to create your own graphics and images based on simple prompt. Approaches such as Large Language Models or the Natural Language Processingwhich are also based on Deep Learning algorithms, are also useful in the content creation.
AI tools
Use the power of artificial intelligence
- Create your website in record time
- Boost your activity thanks to marketing by AI
- Save time and get better results